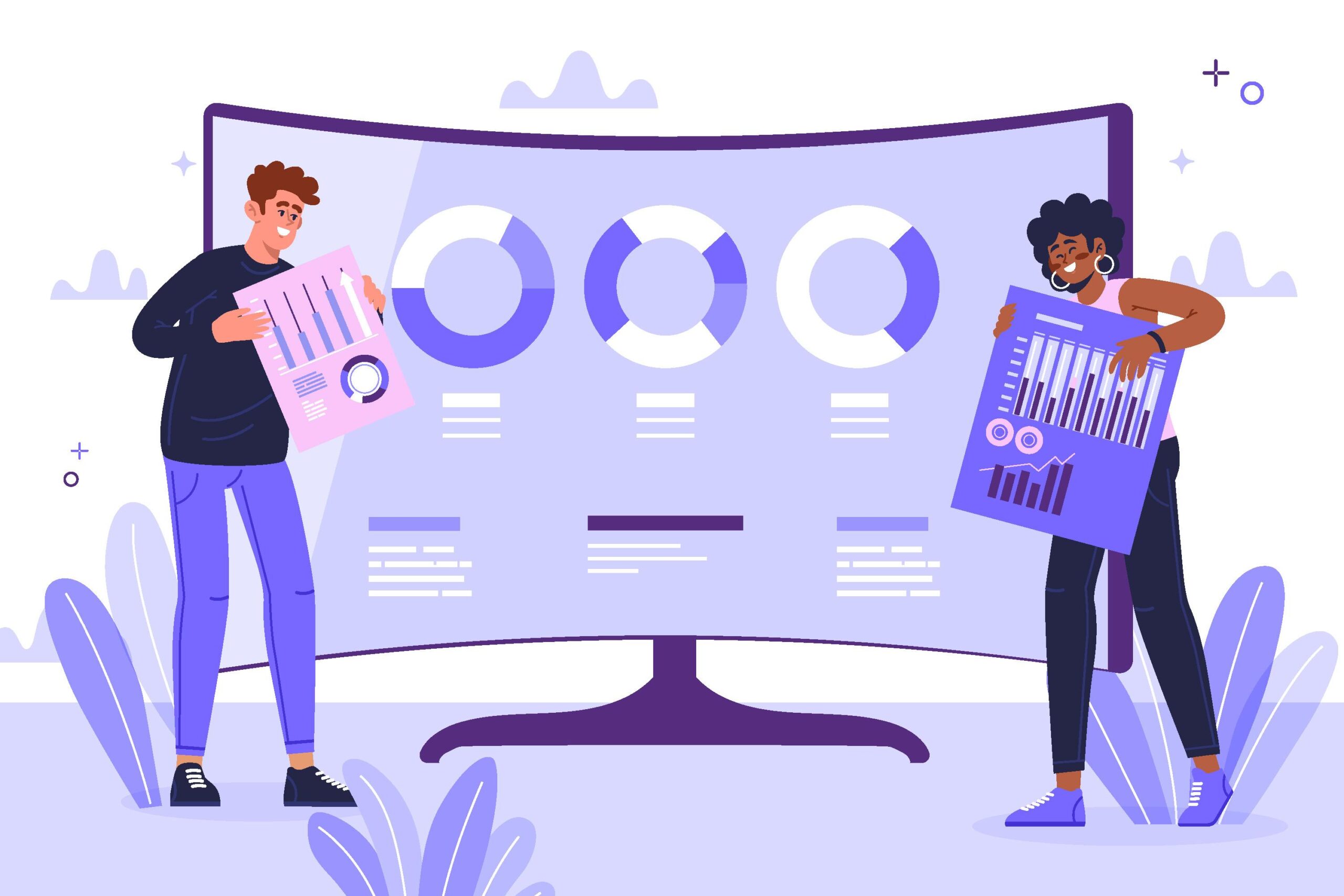
Introduction to Data Labeling Services
Welcome to the world of data labeling services, where precision meets potential across diverse industries! Imagine a digital universe where every piece of information is accurately categorized and annotated for seamless machine learning algorithms. Data labeling services play a pivotal role in this process, ensuring that raw data is transformed into valuable insights. Let’s delve deeper into the realm of data labeling and explore its significance in various sectors.
Importance of Accurate Data Labeling in Various Industries
- Accurate data labeling services is the backbone of various industries today.
- From healthcare to autonomous vehicles, precise data annotation plays a crucial role in ensuring the quality and reliability of machine learning models.
- In healthcare, correctly labeled medical images can assist doctors in making accurate diagnoses and treatment decisions.
- For e-commerce companies, properly labeled product images can enhance search accuracy and boost sales.
- In the financial sector, accurately annotated data helps detect fraudulent activities and manage risks effectively.
- In agriculture, precise labeling of crop images supports farmers in monitoring plant health and maximizing yields.
- The entertainment industry also benefits from accurate data labeling by providing personalized content recommendations to users.
- Accurate data labeling empowers businesses across different sectors to make informed decisions based on reliable insights derived from high-quality annotated datasets.
Common Challenges Faced in Data Labeling Process
Data labeling is a crucial step in preparing data for machine learning algorithms across various industries. However, it comes with its set of challenges. One common issue faced in the data labeling process is ensuring consistency among annotators. Different individuals may interpret labeling instructions differently, leading to inconsistencies in the labeled data.
Another challenge is scalability. As datasets grow larger, manual data labeling becomes time-consuming and costly. Finding efficient ways to scale up the labeling process without compromising accuracy is essential for businesses looking to leverage machine learning effectively.
Quality control also poses a significant challenge in data labeling services. Ensuring that labeled data meets high standards of accuracy requires continuous monitoring and feedback mechanisms. Without robust quality assurance processes in place, errors can propagate through the entire training dataset, impacting model performance.
Addressing these challenges requires implementing stringent guidelines, automated tools, and regular audits to maintain label quality consistently throughout the data annotation process.
Best Practices for Implementing Data Labeling Services
Implementing data labeling services in various industries requires adherence to best practices to ensure accuracy and efficiency. One key practice is establishing clear guidelines and instructions for annotators, providing them with a structured framework for labeling data consistently.
It’s also crucial to regularly evaluate the quality of labeled data through rigorous quality assurance processes. This includes conducting periodic reviews, implementing feedback loops, and addressing any discrepancies promptly.
Utilizing advanced tools and technologies can streamline the labeling process, increasing productivity and reducing human error. Automation techniques such as semi-supervised learning or active learning can optimize resources while maintaining high-quality annotations.
Collaboration among cross-functional teams is essential for successful implementation. Communication channels should be open to address challenges, share insights, and align on objectives throughout the data labeling project lifecycle.
Industry-specific Examples of Successful Data Labeling Implementation
In the healthcare industry, data labeling services have been instrumental in improving the accuracy of medical image analysis. By correctly labeling various elements within images, such as tumors or anomalies, machine learning algorithms can provide more precise diagnoses and treatment plans. In the automotive sector, data labeling plays a crucial role in developing autonomous driving technology. Labeled data helps vehicles recognize objects on the road accurately and make informed decisions in real-time to ensure passenger safety.
E-commerce companies leverage data labeling to enhance personalized recommendations for customers based on their browsing history and preferences. This leads to increased customer satisfaction and higher conversion rates as users are presented with relevant products or services.
Financial institutions use data labeling to detect fraudulent activities by analyzing patterns within labeled transactions. This proactive approach minimizes risks and protects both the institution and its clients from potential security breaches.
These industry-specific examples demonstrate how successful implementation of data labeling services can revolutionize processes, drive innovation, and ultimately lead to improved outcomes across various sectors.
Impact and Benefits of Properly Labeled Data
Properly labeled data plays a crucial role in enhancing the accuracy and efficiency of machine learning algorithms across various industries. By ensuring that data is correctly categorized and annotated, organizations can significantly improve the performance of their AI models. This leads to more precise predictions, better decision-making, and ultimately, increased productivity.
One of the key benefits of properly labeled data is its ability to enhance customer experiences. In sectors like e-commerce and retail, accurate labeling enables personalized recommendations based on user preferences. This not only boosts customer satisfaction but also drives sales and revenue growth.
Furthermore, in healthcare, precisely labeled medical images and patient records help doctors make more accurate diagnoses and treatment plans. This results in improved patient outcomes and overall healthcare quality.
The impact of properly labeled data cannot be underestimated. It’s a foundational pillar for driving innovation, optimizing processes, and achieving competitive advantage in today’s data-driven world.
Future Trends and Innovations in Data Labeling Services
As technology continues to advance at a rapid pace, the future of data labeling company looks promising with several key trends and innovations on the horizon. One major development is the integration of artificial intelligence and machine learning algorithms into data labeling processes, enabling automation and improving efficiency. This shift towards more intelligent systems will not only speed up labeling tasks but also enhance accuracy.
Another trend to watch out for is the rise of specialized data labeling platforms tailored to specific industries such as healthcare, autonomous vehicles, and e-commerce. These platforms will offer industry-specific tools and workflows to address unique labeling requirements effectively. Additionally, we can expect a greater emphasis on data privacy and security measures in data labeling services to ensure compliance with regulations like GDPR.
Furthermore, collaborative crowdsourcing approaches are likely to become more prevalent in the future as companies tap into global talent pools for large-scale annotation projects. The combination of human annotators and AI-powered tools will continue to drive innovation in data labeling methodologies, paving the way for more precise training datasets across various sectors.
Conclusion
Data labeling services play a crucial role in various industries by ensuring accurate and high-quality labeled data for machine learning algorithms. Implementing best practices such as using advanced tools, training labelers effectively, and monitoring quality control can significantly improve the efficiency of the data labeling process. The impact of properly labeled data is evident in improved model accuracy, enhanced decision-making processes, and increased operational efficiencies across industries. As technology continues to evolve, future trends in data labeling services are expected to focus on automation, AI-assisted labeling tools, and real-time feedback mechanisms to further enhance the quality and speed of data labeling tasks. By embracing these innovations and industry-specific strategies for successful implementation of data labeling services, organizations can unlock new opportunities for growth and innovation in the era of big data analytics.