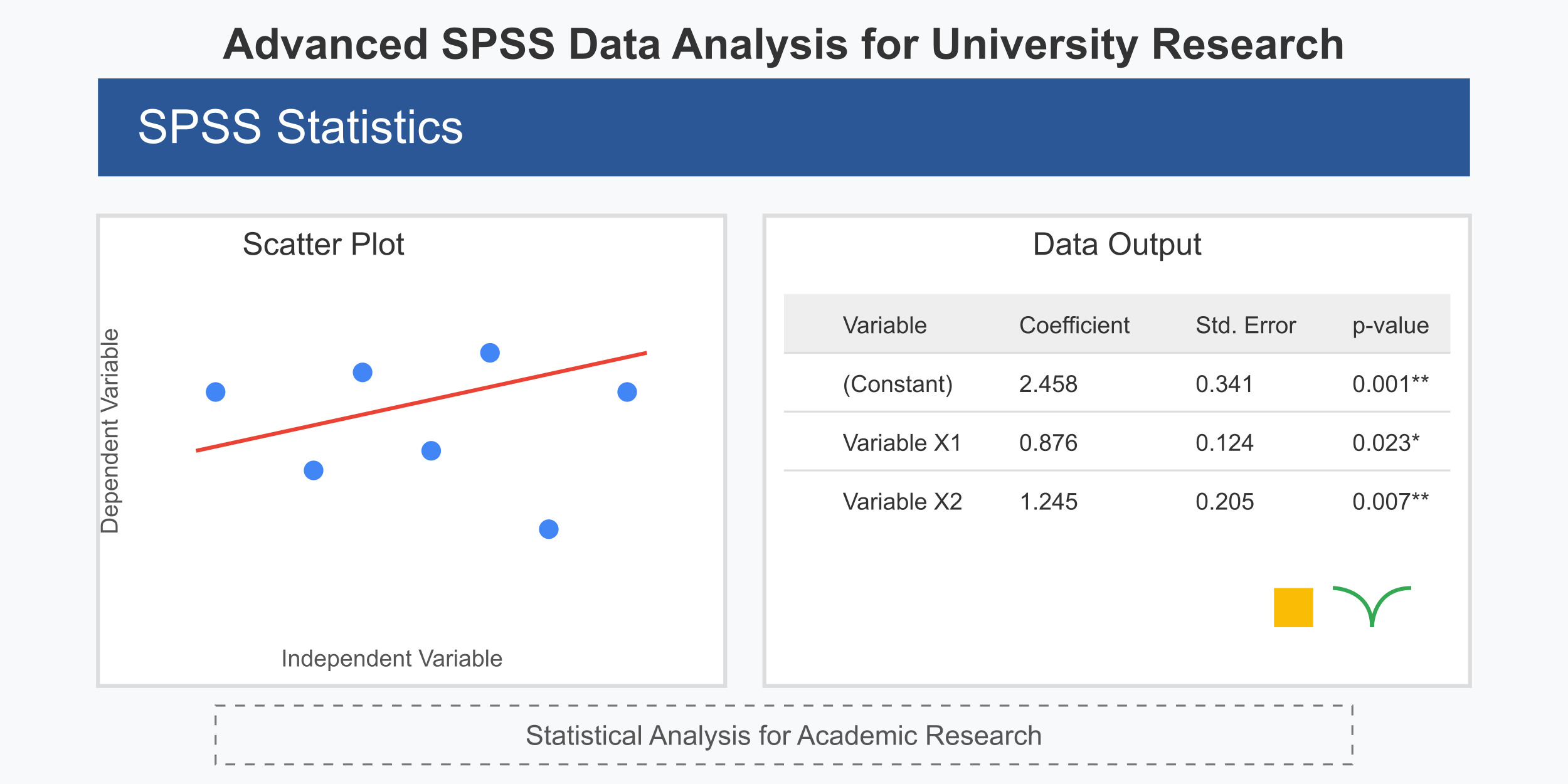
Alright, so you’re diving into social science research, huh? And now you gotta figure out whether to use a parametric or a non-parametric test in SPSS. Don’t sweat it! This stuff can sound all fancy and intimidating, but I promise you—it’s not rocket science. Well, maybe a little science, but nothing you can’t handle.
The Basics: What’s the Deal?
When you’re crunching numbers in SPSS (or any stats software), the type of test you pick depends on what kinda data you’re working with. Parametric tests? They’re the VIPs of stats world—assuming all the right conditions, following normal distribution, and making life easy when the data behaves. Non-parametric tests? More like the wildcards. They don’t need all those fancy assumptions and can handle messy, wonky data like a champ.
Think of it like baking cookies. Parametric tests are like using a recipe to the T—measuring every ingredient, setting the perfect oven temp, and getting predictable, golden-brown deliciousness. Non-parametric tests? More like eyeballing ingredients and hoping for the best, but somehow, they still turn out just fine.
When to Go Parametric
Okay, so parametric tests are kinda picky. They assume your data is normally distributed, which means your data should have that classic bell-curve shape. If your data fits the bill, parametric tests are the way to go because they’re more powerful. That means they detect effects more easily when those effects actually exist.
Some popular parametric tests in SPSS:
- T-tests – Comparing two groups? Like seeing if students who pull all-nighters score worse than those who sleep? That’s a t-test for ya.
- ANOVA (Analysis of Variance) – This is like a t-test but for three or more groups. Maybe you wanna see if freshmen, sophomores, and seniors all have different levels of stress? Boom, ANOVA.
- Pearson’s Correlation – Wanna see if two variables are related, like caffeine intake and energy levels? Pearson’s gotcha.
- Regression Analysis – If you’re trying to predict an outcome based on one or more variables (like, does social media use predict self-esteem?), regression is your friend.
When to Ditch Parametric and Go Non-Parametric
Now, sometimes your data just won’t play nice. Maybe it’s skewed, has outliers, or is just plain weird. Or maybe you have a small sample size, and the normality assumption goes out the window. That’s when you bring in the non-parametric tests—they don’t care about normality and can handle all the statistical chaos.
Here are some common ones:
- Mann-Whitney U Test – Like a t-test but for non-normal data. Maybe you wanna compare two groups, like social media users vs. non-users on happiness levels, but your data’s all over the place.
- Kruskal-Wallis Test – Like an ANOVA but for non-parametric data. Testing stress levels across multiple college majors but your data isn’t normal? This test has your back.
- Spearman’s Rank Correlation – Similar to Pearson’s, but for data that doesn’t follow the normal curve. Perfect when your relationship looks more like a wonky rollercoaster than a straight line.
- Chi-Square Test – Great for categorical data. If you’re looking at whether pet owners vs. non-pet owners have different exercise habits, this one’s gold.
A Quick Side Tangent: Normality Tests
Before you decide between parametric or non-parametric, ya gotta check if your data is normal. In SPSS, you can run tests like the Kolmogorov-Smirnov or Shapiro-Wilk to see if your data behaves itself. But honestly? With big sample sizes, these tests get super sensitive. Sometimes, just looking at histograms and boxplots does the trick. Trust your gut!
Real-World Example (Because Who Likes Dry Theory?)
Let’s say you’re researching for SPSS homework on whether coffee drinkers are happier than tea drinkers. You survey 50 people, split ‘em into two groups, and measure their happiness levels. If your data looks nice and normal? Run a t-test. But if the data’s wonky—like some folks are off-the-charts happy while others are total grumps—then Mann-Whitney U is your best bet.
And hey, while we’re on the subject—if you’re analyzing coffee drinkers, don’t forget to check their sleep patterns. Because let’s be real, coffee might be making them think they’re happier while secretly running them into the ground. But hey, that’s another study for another day.
Final Thoughts (And a Little Pep Talk)
So there you have it—parametric vs. non-parametric tests in SPSS, without all the scary math jargon. If your data’s nice and normal, stick with parametric. If it’s messy and unpredictable, go non-parametric. And don’t stress too much—stats might seem intimidating, but with a little practice, you’ll be running tests like a pro.
Oh, and one last thing—SPSS might seem kinda clunky, but once you get the hang of it, it’s not so bad. Just don’t forget to save your work. Nothing’s worse than running a perfect analysis only for SPSS to crash and burn (been there, done that—never again).