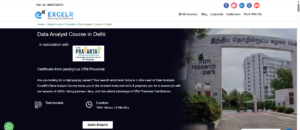
data analytics institute in Delhi
Introduction
Text analytics is a great tool to extract insights out of unstructured data, such as text documents, social media posts, emails, and more. Unstructured data needs to be prepared for analysis. This involves cleaning the data, and organising, tokenising, and classifying text before analysing it. This article sheds some light on the basic aspects of text analytics that are covered in the course curriculum of any data analytics institute in Delhi.
Text Analytics Basics
Here is a basic overview of how text analytics works and some common techniques used:
- Text Preprocessing:
This step involves cleaning the text data and preparing it for analysis. It typically includes tasks like removing punctuation, converting text to lowercase, removing stopwords (common words like “and,” “the,” “is,” and so on), and stemming or lemmatising words (reducing them to their base form).
- Tokenisation:
Tokenisation is the process in which the text is broken down into smaller units, such as words or sentences. This step is crucial for further analysis because it helps to identify the basic elements of the text.
- Named Entity Recognition (NER):
A technique used to detect and classify named entities mentioned in the text, such as people’s names, organisations, locations, dates, and so on, is NER. This is highly useful for tasks like extracting key information from documents or understanding the relationships between entities. This is a key capability sought by data analysts working in commercialised cities where businesses need to handle large volumes of data. To cater to this demand, a data analyst training in commercialised cities would mostly cover NER as a focus topic.
- Sentiment Analysis:
This technique aims to determine the opinion expressed or underlying sentiment in a piece of text. It can classify the sentiment as positive, negative, or neutral, and sometimes provide a sentiment score indicating the intensity of the sentiment. Sentiment analysis is predominantly used in social media monitoring, customer feedback analysis, and market research. Urban marketing professionals need to be innovative and must evolve novel customer-facing strategies to meet their targets. Sentiment analysis has immense potential to expose customer preferences and trends. For this reason, a data analytics institute in Delhi, Chennai, or Bangalore that offers courses designed for marketing professionals would include detailed studies in sentiment analysis.
- Topic Modelling:
Topic modelling is used to identify topics or themes present in a document collection. It automatically deciphers the underlying structure of the text data and assigns a distribution of topics to each document. Popular algorithms for topic modelling include Latent Dirichlet Allocation (LDA) and Non-Negative Matrix Factorisation (NMF).
- Text Classification:
Text classification involves categorising text documents into predefined categories or classes. This can be done using ML algorithms such as Support Vector Machines (SVM), Naive Bayes, or deep learning models like Convolutional or Recurrent Neural Networks. Text classification is used for tasks like spam detection, sentiment analysis, and content categorisation.
- Keyword Extraction:
Keyword extraction involves identifying the most important or relevant words or phrases from a piece of text. This can help summarise the main topics discussed in the text or identify key terms for further analysis.
- Word Embeddings:
Word embeddings are dense vector representations of words in a high-dimensional space, where similar words are close to each other. Techniques like Word2Vec, GloVe, and FastText are commonly used to learn word embeddings from large text corpora. Word embeddings capture semantic relationships between words and are often used as features in various natural language processing tasks.
Summary
These are just some of the basic techniques used in text analytics. Depending on the specific goals and project requirements, additional techniques and tools may be employed to extract valuable insights from unstructured text data. Before enrolling for a course that covers text analytics, go through the course curriculum and ensure that it is oriented for meeting the specific requirements of your professional role.